This may well be a sobering year when it comes to AI adoption, use and scaling. On the demand side, organizations will be pulling investments back prematurely because they’re not seeing the value they expected. On the supply side, supply shortages, unmet expectations and investor pressure have caused one big tech company to reduce AI infrastructure investments and others will follow, according to Forrester.
To date, organizations have been investing heavily in AI and GenAI, not necessarily with a view toward ROI, though ROI can be difficult to quantify from a hard dollar perspective, which senior executives and boards now want. The anticipated shortage of infrastructure will also likely have an impact.
What’s Happening on the Demand Side
Organizations will not continue to increase investments in AI if they’re not seeing the value they expect.
“[C]ompanies are scaling back on their AI investments or too impatient in terms of ROI. They will [likely] scale back on their AI investment prematurely, which is not a good strategy,” says Jayesh Chaurasia, analyst at Forrester. “The other factor that might be fueling this is the current economic climate. In the last three months, almost everyone is trying to cut back on any type of investment that is not generating a clear ROI, and not only the AI-related stuff.”
Executives are asking for ROI numbers on analytics, data governance, and data quality programs, and they are demanding dollar values as opposed to “improving customer experience” or “increasing operational efficiency.”
“In 2023 and this year too, we are seeing more focus on ROI related to generative AI,” says Chaurasia. “Almost every executive was talking about how generative AI is going to just change the world, but it’s not as easy as just deploying a model or a generated AI function and then say your job is done because there is a foundational data analytics requirement that will eventually enable it, and which means you need to have proper privacy and security protocols, [such as] access management and data governance. You also must supply better data quality [because] these models are trained on the entire data set from the internet.”
The fact that people know the models are trained on internet data has inspired internet postings that are intentionally inaccurate or misleading, so the models won’t work right.
“The better answer is, of course, to use your own industry enterprise data, which gives the AI model more information about your company,” says Chaurasia. “You can very easily set up a connection with your data warehouse and get all the data into the model, but it’s not that easy because privacy, security, and governance are not in place. So, you’re not 100% sure whether you’re sharing your data with the model or the entire world.”
Organizations have expected quick returns but not realized them because the initial expectations were unrealistic. Later comes the realization that the proper foundation has not been put in place.
“Folks are saying they expect ROI in at least three years and more than 30% or so are saying that it would take three to five years when we’ve got two years of generative AI. [H]ow can you expect it to perform so quickly when you think it will take at least three years to realize the ROI? Some companies, some leadership, might be freaking out at this moment,” says Chaurasia. “I think the majority of them have spent half a million on generative AI in the last two years and haven’t gotten anything in return. That’s where the panic is setting in.”
Explaining ROI in terms of dollars is difficult, because it’s not as easy as multiplying time savings by individual salaries. Some companies are working to develop frameworks, however.
“Some managers are reaching out to every business unit to ask the benefits that they have received with proper understanding of ownership, where the data exists [and] lineage of particular data set. They are using some custom surveys to reach out to all the employees in the organization to for their suggestions as well as their metrics,” says Chaurasia. “Unfortunately, there is no single framework that I would suggest works for every company.”
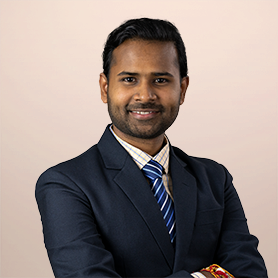
Jayesh Chaurasia, Forrester
Chaurasia is working on KPIs for the various domains, in terms of quality, governance, MDM, data management, data storage and everything that companies can track over the time to see the improvement, but they’re not connected to dollar value.
“What I’m recommending is find at the tactical, managerial, and executive levels what matters to them [and have] KPIs for each of those different layer levels to maintain and calculate that ROI regularly, so that they can use that KPI those metrics to show the benefit of whether they have improved over time or not.”
View From the Supply Side
If enterprises are reducing AI investments because the anticipated benefits aren’t being realized, vendors will pull back. Meanwhile, China has banned the export of critical materials required for semiconductors and other tech-related technologies in response to President-elect Donald Trump’s planned tariffs, not to mention the downstream impacts of tariffs — higher production costs and therefore higher tech prices IT departments will have to bear when budgets are already tight and may become tighter.
Bottom Line
Infrastructure shortages due to reduced AI investments on the demand side combined with higher prices and a potential US chip shortage due to lack of materials on the supply side would in turn impact the calculus of AI ROI. There are also broader impacts of the incoming administration’s policies such as mass deportation, which could impact tech workers, including AI talent, and their employers.