FICO Chief Analytics Officer Scott Zoldi has spent the last 25 years at HNC and FICO (which merged) leading analytics and AI at HNC FICO is well known in the consumer sector for credit scoring, while the FICO Platform helps businesses understand their customers better so they can provide hyper-personalized customer experiences.
“From a FICO perspective, it’s making sure that we continue to develop AI in a responsible way,” says Zoldi. “There’s a lot of [hype] about generative AI now and our focus has been around operationalizing it effectively so we can realize this concept of ‘the golden age of AI’ in terms of deploying technologies that actually work and solve business problems.”
While today’s AI platforms make model governance and efficient deployment easier, and provide greater model development control, organizations still need to select an AI technique that best fits the use case.
A lot of the model hallucinations and unethical behavior are based on the data on which the models are built, Zoldi says. “I see companies, including FICO, building their own data sets for specific domain problems that we want to address with generative AI. We’re also building our own foundational models, which is fully within the grasp of almost all organizations now,” he says.
He says their biggest challenge is that you can never totally get rid of hallucinations. “What we need to do is basically have a risk-based approach for who’s allowed to use the outputs, when they’re allowed to use the outputs, and then maybe a secondary score, such as a AI risk score or AI trust score, that basically says this answer is consistent with the data on which it was built and the AI is likely not hallucinating.”
Some reasons for building one’s own models include full control of how the model is built, and reducing the probability of bias and hallucinations based on the data quality.
“If you build a model and it produces an output, it could be hallucination or not. You won’t know unless you know the answer, and that’s really the problem. We produce AI trust scores at the same time as we produce the language models because they’re built on the same data,” says Zoldi. “[The trust score algorithms] understand what the large language models are supposed to do. They understand the knowledge anchors — the knowledge base that the model has been trained on — so when a user asks a question, it will look at the prompts, what the response was, and provide a trust score that indicates how well aligned the model’s response is aligned with the knowledge anchors on which the model was built. It’s basically a risk-based approach.”
FICO has spent considerable time focused on how to best incorporate small or focused language models as opposed to simply connecting to a generic GenAI model via an API. These “smaller” models may have eight to 10 billion parameters versus 20 billion or more than 100 billion, for example.
He adds that you can take a small language model and achieve the same performance of a much larger model, because you can allow that small language model to spend more time reasoning out an answer. “And it’s powerful because it means that organizations that can only afford a smaller set of hardware can build a smaller model and deploy it in such a way that it’s less costly to use and just as performant as a large language model for a lot less cost, both in model development and in the inference costs of actually using it in a production sense.”
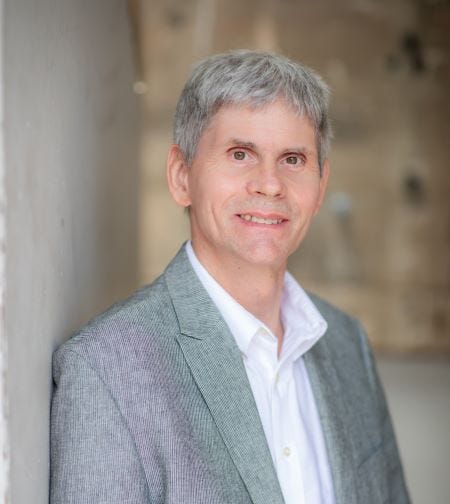
Scott Zoldi
The company has also been using agentic AI.
“Agentic AI is not new, but we now have frameworks that assign decision authority to independent AI operators. I’m okay with agentic AI, because you decompose problems into much simpler problems, and those simpler problems [require] much simpler models,” says Zoldi. “The next area is a combination of agentic AI and large language models, though building small language models and solving problems in a safe way is probably top of mind for most of our customers.”
For now, FICO’s primary use case for agentic AI is generating synthetic data to help counter and stay ahead of threat actors’ evolving methods. Meanwhile, FICO has been building focused language models that address financial fraud and scams, credit risks, originations, collections, behavior scoring and how to enable customer journeys. In fact, Zoldi recently created a focused model in only 31 days using a very small GPU.
“I think we’ve all seen the headlines about how these humongous models with billions of parameters and thousands of GPUs, but you can go pretty far with a single GPU,” says Zoldi.
Challenges Zoldi Sees in 2025
One of the biggest challenges CIOs faces is anticipating the shifting nature of the US regulatory environment. However, Zoldi believes regulation and innovation go hand in hand.
“I firmly believe that regulation and innovation inspire each other, but others are wondering how to develop their AI applications appropriately when [they’re not prescriptive],” says Zoldi. “If they don’t tell you how to meet the regulation, then you’re guessing how the regulations might change and how to meet them.”
Many organizations consider regulation a barrier to innovation rather than an inspiration for it.
“The innovation is basically a challenge statement like, ‘What does that innovation need to look like?’ so that I can meet my business objective, get a prediction, and have an interpretable model while also having ethical AI. That means better models,” says Zoldi. “Some people believe there shouldn’t be any constraints, but if you don’t have them, people will continue to ask for more data and ignore copyrights. You can also go down a deep learning path where models are uninterpretable, unexplainable, and often unethical.”
What Innovation at FICO Looks Like
At FICO, innovation and operationalization are synonymous.
“We just built our first focused model last year. We’ve been demonstrating how small models on task specific domain problems perform just as well as large language models you can get commercially, and then we operationalize it,” says Zoldi. “That means I’m coming up with the most efficient way to embed AI in my software. We’re looking at unique software designs within our FICO Platform to enable the execution of these technologies efficiently.”
Some time ago, Zoldi and his team wanted to add audit capabilities to the FICO Platform. To do it, they used AI blockchains.
“An AI blockchain codifies how the model was developed, what needs to be monitored, and when you pull the model. Those are really important concepts to incorporate from an innovation perspective when we operationalize, so a big part of innovation is around operationalization. It’s around the sensible use of generative AI to solve very specific problems in the pockets of our business that would benefit most. We’re certainly playing with things like agentic AI and other concepts to see whether that would be the attractive direction for us in the future.”
The audit capabilities FICO built can track every decision made on the platform, what decisions or configurations have changed, why they changed, when they changed and who changed them.
“This is about software and the components, how strategies change, and how that model works. One of the main things is ensuring that there is auditing of all the steps that occur when an AI or machine learning model gets deployed in a platform, and how it’s being operated so you can understand things like who’s changing the model or strategy, who made that decision, whether it was tested prior to deployment and what the data is to support the solution. For us, that validation would belong in a blockchain so there is the immutable record of those configurations.”
FICO uses AI blockchains when it develops and executes models, and to memorialize every decision made.
“Observability is a huge concept in AI platforms today. When we develop models, we have a blockchain that explains how we develop it so we can meet governance and regulatory requirements. On the same blockchain, are exactly what you need for real-time monitoring of AI models, and that wouldn’t be possible if observability was not such a core concept in today’s software,” says Zoldi. “Innovation in operationalization really comes from the fact that the software on which organizations build and deploy their decision solutions are changing as software and cloud computing advance, so the way we would have done it 25, 20, or 10 years ago is not the way that we do it most efficiently today. And that changes the way that we must operationalize. It changes the way we deploy and the way we even look at basic things like data.”
Why Zoldi Has His Own Software Development Team
Most software development organizations fall under a CIO or CTO, which is also true at FICO, though Zoldi also has his own software development team and works in partnership with FICO’s CTO.
“If a FICO innovation has to be operationalized, there must be a near term view to how it can be deployed. Our software development team makes sure that we come up with the right software architectures to deploy because we need the right throughput and latency,” says Zoldi. “Our CTO, Bill Waid, and I both focus a lot of our time on what are those new software designs so that we can make sure that all that value can be operationalized.”
A specialized software team has been reporting to Zoldi for nearly 17 years, and one benefit is that it allows Zoldi to explore how he wants to operationalize, so he can make recommendations to the CTO and platform teams and ensure that new ideas can be operationalized responsibly.
“If I want to take one of these focus language models and understand the most efficient way to deploy it and do inferencing, I’m not dependent on another team. It allows me to innovate rapidly, because everything that we develop in my team needs to be operationalized and be able to be deployed. That way, I don’t come with just an interesting algorithm and a business case. I come with an interesting algorithm, a business case and a piece of software so I can say these are the operating parameters of it. It allows me to make sure that I essentially have my own ability to prioritize where I need software talent focused from my types of problems for my AI solutions. And that’s important because, I may be looking three years, four, or five years ahead, and need to know what we will need.”
The other benefit is that the CTO and the larger software organization don’t have to be AI experts.
“I think most high performing AI machine learning research teams like the one that I run, really need to have that software component so they have some control, and they’re not in some sort of prioritization queue for getting some software attention,” says Zoldi. “Unless those people are specialized in AI, machine learning and MLOps, it’s going to be a poor experience. That’s why FICO is taking this approach and why we have the division of concerns.”