Artificial Intelligence is virtually everywhere, whether enterprises have an AI strategy or not. As AI capabilities continue to get more sophisticated, businesses are trying to capitalize on it, but they haven’t done enough foundational work to succeed. While it’s true that companies have been increasing their AI budgets over the last several years, it’s become clear that the ROI of such efforts varies significantly, based on many dynamics, such as available talent, budget, and a sound strategy. Now, organizations are questioning the value of such investments to the point of pulling back in 2025.
According to Anand Rao, distinguished service professor, applied data science and artificial Intelligence at Carnegie Mellon University, the top three challenges are ROI measurement, realization, and maintenance.
“If the work I’m doing takes three hours and now it takes a half an hour, that’s easily quantifiable, [but] human performance is variable,” says Rao. “The second way is having a baseline. We don’t [understand] human performance, but we are saying AI is 95% better than a human, but which human? The top-most performer, an average performer, or the new employee?”
When it comes to realizing ROI, there are different ways to look at it. For example, if AI saves 20% of five peoples’ time, perhaps one could be eliminated. However, if those five people are now spending more time on higher value tasks, then it would be unwise to let any of them go because they are providing more value to the business.
The other challenge is maintenance because AI models need to be monitored and maintained to remain trustworthy. Also, as humans use AI more frequently, they get more adept at doing so while AI is learning from the human, which may increase performance. Enterprises are not measuring that either, Rao says.
“[T]here’s a whole learning curve happening between the human and the AI, and independently the two. That might mean that you may not be able to maintain your ROI, because it may increase or decrease from the base point,” says Rao.
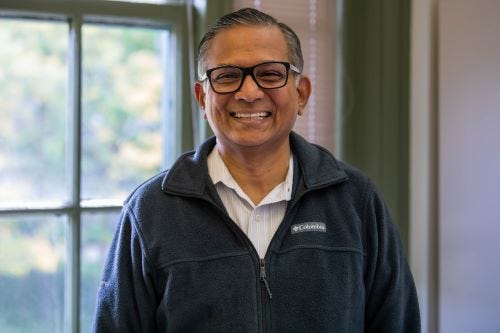
Anand Rao, Carnegie Mellon University
There’s also a time element. For example, ChatGPT-4 was introduced in March 2023, but enterprises weren’t ready for it, but in six months or less, businesses had started investing systematically to develop their AI strategy. Nevertheless, there’s still more to do.
[T]he crucial fact is that we are still in the very early days of this technology, and things are moving very quickly,” says Beatriz Sanz Saiz, global consulting data and AI Leader at business management consulting firm EY. “Enterprises should become adept at measuring value realization, risk and safety. CIOs need to rethink a whole set of metrics because they will need to deliver results. Many organizations have a need for a value realization office, so that for everything they do, they can establish metrics upfront to be measured against, whether that is cost savings, productivity, new revenue growth, market share, employee satisfaction [or] customer satisfaction.”
The GenAI Impact
While many enterprises have had plenty of success with traditional AI, Kjell Carlsson, head of AI strategy at enterprise MLOps platform Domino Data Lab, estimates that 90% of GenAI initiatives are not delivering results that move the needle on a sustained basis, nor are they on track to do so.
“[M]ost of these organizations are not going after use cases that can deliver transformative impact, nor do they have the prerequisite AI engineering capabilities to deliver production-grade AI solutions,” says Carlsson. “Many organizations are under the misconception that merely making private instances of LLMs and business apps with embedded GenAI capabilities available to business users and developers is an effective AI strategy. It is not. While there have been productivity gains from these efforts, in most cases, these have been far more modest than expected and have plateaued quickly.”
Though GenAI has many similarities to driving business value with traditional AI and machine learning, it requires expert teams that can design, develop, operationalize and govern AI applications that rely on complex AI pipelines. These pipelines combine data engineering, prompt engineering, vector stores, guardrails, upstream and downstream ML and GenAI models, and integrations with operational systems.
“Successful teams have evolved their existing data science and ML engineering capabilities into AI product and AI engineering capabilities that allow them to build, orchestrate and govern extremely successful AI solutions,” says Carlsson.
.jpg?width=400&auto=webp&quality=80&disable=upscale)
Kjell Carlsson, Domino Data Lab
Sound tech strategies identify a business problem and then select the technologies to solve it, but with GenAI, users have been experimenting before they define a problem to solve or expected payoff.
“[W]e believe there is promise of transformation with AI, but the practical path is unclear. This shift has led to a lack of focus and measurable outcomes, and the derailment of plenty of AI efforts in the first wave of AI initiatives,” says Brian Weiss, chief technology officer at hyperautomation and enterprise AI infrastructure company Hyperscience. “In 2025, we anticipate a more pragmatic or strategic approach where generative AI tools will be used to deliver value by attaching to existing solutions with clearly measurable outcomes, rather than simply generating content. [T]he success of AI initiatives hinges on a strategic approach, high-quality data, cross-functional collaboration and strong leadership. By addressing these areas, enterprises can significantly improve their chances of achieving meaningful ROI from their AI efforts.”
Andreas Welsch, founder and chief AI strategist at boutique AI strategy firm Intelligence Briefing, says early in the GenAI hype cycle, organizations were quick to experiment with the technology. Funding was made available, and budgets were consolidated to explore what the technology could offer, but they didn’t need to deliver ROI. Times have changed.
“Organizations who have been stuck in the exploration phase without assessing the business value first, are now caught off guard when the use case does not deliver a measurable return,” says Welsch. “Set up a formal process and governance that assess the business value and measurable return of an AI product or project prior to starting. Secure stakeholder buy-in and establish a regular cadence to measure progress, ensure continued support or stop the project, [and] assess existing applications in your company. Which of those offers AI capabilities that you are not using yet? You don’t need to build every app from scratch.”
Many Potholes to Navigate
Jamie Smith, CIO at University of Phoenix, says the cost of AI is being reflected more frequently in SaaS contracts, whether the contracts specify it or not.
“We’ve seen this in the past 6 months, as the cost to compute using AI rises and rises and is set to continue to do so as models grow more robust — and therefore more power hungry. SaaS providers are looking at their utility bills and passing the cost to businesses,” says Smith. “As a result, SaaS contracts — and partnerships more broadly — are going to come under a lot more scrutiny. If these costs are rising, then partners’ productivity needs to match.”
Edward Smyshliaiev, chief technology officer at Hedgefun:D says many organizations derail their AI ROI though a combination of overambition, under-preparation and a lack of alignment between AI teams and business leaders.
“AI isn’t a magic wand; it’s a tool. To wield it effectively, companies need to ensure data pipelines are clean and reliable and invest in training staff to interpret and act on AI outputs,” says Smyshliaiev. “A shared vision between AI teams and leadership is critical — everyone must know what success looks like and how to measure it.”
Sean Bhardwaj, managing partner at strategic consulting firm Breakthrough Growth Partners is a fractional chief AI officer and strategist. In this role, he’s observed that two of the top reasons enterprises aren’t realizing better ROI on their AI initiatives is because they lack a foundational strategy and focus on the human side of AI adoption.
For example, one of his clients wanted to implement AI-driven customer recommendations, only to discover mid-project that the data infrastructure couldn’t support it. Similarly, organizations often assume that teams will adopt AI enthusiastically, which isn’t necessarily the case.
“Planning for adoption with training and incentives is essential to see real engagement and impact, says Bhardwaj. “I advise companies to see each stage as an investment in capability-building, with each phase laying the groundwork for the next.”
All too often, organizations discard AI initiatives that don’t meet initial expectations rather than rethinking their approach.
John Bodrozic, co-founder and CIO at homeowner lifecycle platform HomeZada, has observed that enterprises are relying solely on standalone AI to solve problems or find new growth opportunities, but they are ultimately being led by development teams and not product management teams.
“There are so many areas where AI can impact bottom-line cost savings and top line revenue growth, but only when these use-case scenarios are explored by cross-functional teams that combine software and AI development specialists with members of the functional team,” says Bodrozic. “Without this direct interaction, ROI from AI is challenging at best.”
The Business View
A 2023 Gartner report found that only 54% of AI projects get past the proof-of-concept phase, and many of those fail to deliver on the promised financial or operational impact. According to Ed Gaudet, CEO and founder of health care risk management solution provider Censinet, companies may believe that “AI will make everything better,” but they never specify what “better” means.
“Enterprises must take a phased, strategic approach [that requires] defining clear use cases that have actual business value like the automation of a drudgery, supply chain optimization, or leveraging chatbots to meet better customer experience. Secondly, organizations need to create structural capabilities like a good data governance framework, scalable infrastructure and strong developer and engineering skills. Companies that train their employees in AI have a 43% higher success rate deploying AI projects.”
Nicolas Mougin, consulting and support director at global cloud platform Esker, credits rushed implementations as a reason for ROI shortfalls.
“The pressure to stay competitive in a rapidly evolving technological landscape drives many organizations to implement AI without sufficient planning. Instead of conducting thorough needs assessments or piloting solutions, businesses often rush to deploy tools in the hope of gaining an edge,” says Mougin. “However, hastily executed projects overlook key considerations such as data readiness, scalability or user adoption.”
Edward Starkie, director, GRC at global risk intelligence company Thomas Murray, believes that most organizations are not in a suitable position to be able to adopt AI and exploit it to its fullest extent.
“To be successful there is a level of maturity that is required which [depends] upon having the necessary mechanisms supporting the design, creation and maintenance of the technology in a field which is short of genuine expertise,” says Starkie. “[E]specially at board level, a lack of education is a key contributing factor. [Mandates] are being issued without the without understanding the importance of the core components being in place.”